The Impact of Machine Learning Data Models on Business Success
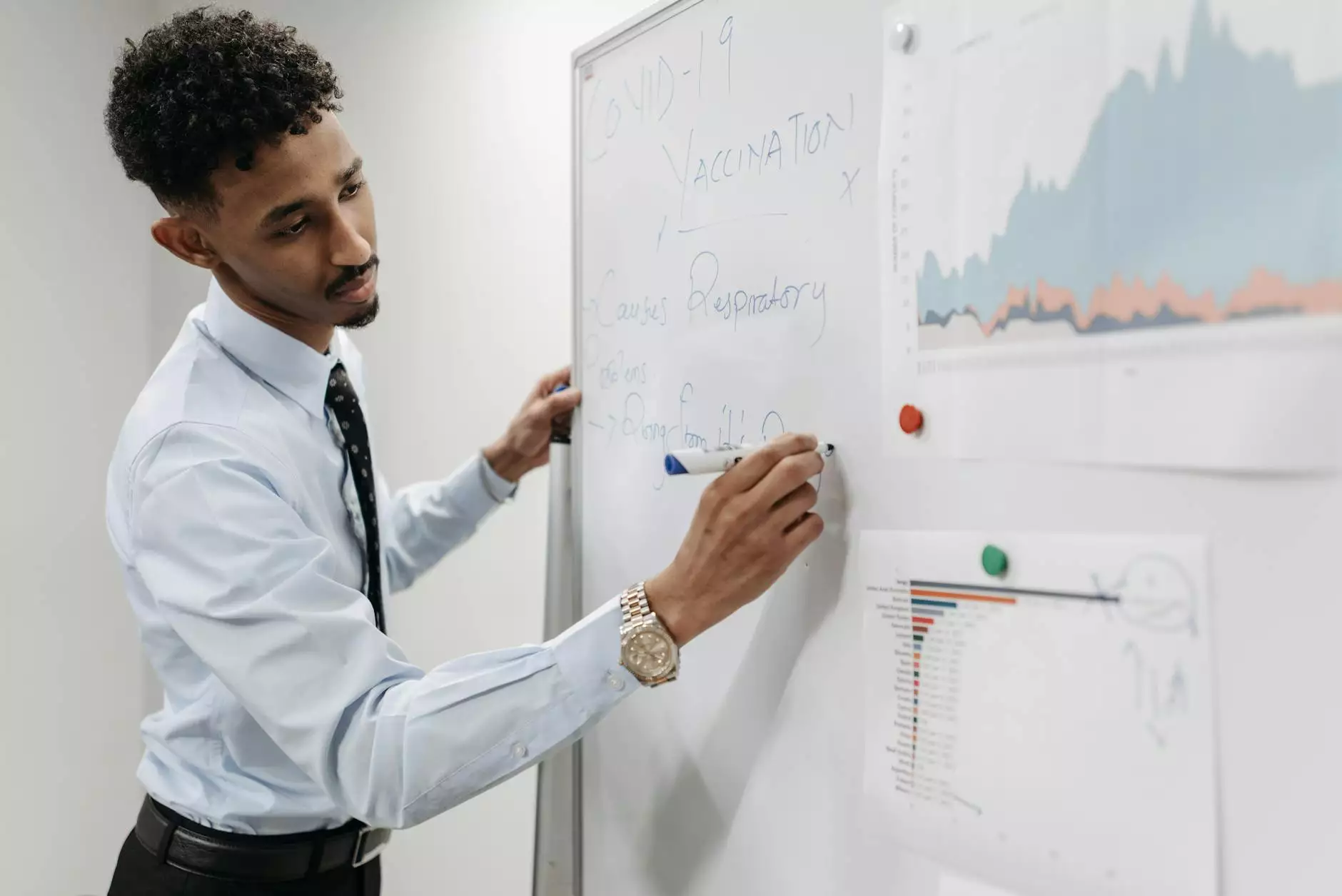
In today's rapidly evolving digital landscape, businesses are increasingly turning to advanced technologies such as machine learning data models to gain a competitive edge. Understanding how these models integrate within various business frameworks—from content management services to business process automation services and data governance systems—is crucial for any organization looking to thrive in the ages of AI and big data.
Understanding Machine Learning Data Models
Machine learning is a subset of artificial intelligence (AI) that enables systems to learn from data, identify patterns, and make decisions with minimal human intervention. Data models serve as the backbone of machine learning applications, transforming raw data into insights that drive actionable outcomes. These models are designed to recognize trends, predict future events, and optimize business processes by analyzing vast amounts of information efficiently.
The Significance of Content Management Services
Content management services (CMS) are essential for businesses that prioritize effective communication and seamless information sharing. By integrating machine learning data models, organizations can enhance their content strategy in several ways:
- Personalization: Machine learning algorithms analyze user behaviors and preferences, allowing businesses to deliver tailored content to individual users, which significantly increases engagement.
- Content Optimization: Through predictive analytics, businesses can ascertain which types of content resonate most with their audience, enabling them to curate effective marketing campaigns.
- Automation: Machine learning can automate various content processes, from tagging and categorizing to automated content creation, freeing up resources for other strategic initiatives.
Business Process Automation Services
Business process automation (BPA) refers to the use of technology to execute recurring tasks or processes in a business where manual effort can be replaced. Incorporating machine learning data models into BPA can streamline operations and improve efficiency:
- Predictive Maintenance: Machine learning models can analyze historical data to predict equipment failures and schedule maintenance proactively, reducing downtime and repair costs.
- Workflow Optimization: By analyzing workflow patterns, machine learning can identify bottlenecks and provide recommendations for improving process efficiency.
- Resource Allocation: Machine learning models help in forecasting demand and optimizing resource allocation, ensuring that businesses operate at peak efficiency.
The Role of Data Governance Systems
As organizations increasingly rely on data to drive decisions, implementing effective data governance systems becomes essential. These systems ensure data integrity, security, and compliance. Machine learning can bolster data governance in the following ways:
- Data Quality Improvement: Machine learning algorithms can identify anomalies and outliers in data sets, prompting data cleansing processes that enhance overall data quality.
- Automated Compliance Monitoring: Leveraging machine learning for compliance ensures that businesses adhere to regulations by automatically monitoring and reporting on data usage and access patterns.
- Enhanced Data Security: By employing machine learning, organizations can detect unusual access patterns and potential security breaches in real-time, allowing for a swift response to threats.
The Convergence of Machine Learning and Business Strategies
To effectively leverage machine learning data models, organizations must align these technologies with their business strategies. Here are several best practices to incorporate machine learning into your business:
- Define Clear Objectives: Before implementing machine learning, clearly define what you hope to achieve—be it increased customer retention, improved operational efficiencies, or enhanced product recommendations.
- Invest in Quality Data: The performance of machine learning models relies heavily on the quality of the input data. Ensure that your data is accurate, comprehensive, and relevant.
- Foster a Data-Driven Culture: Encourage a culture where data-driven decision-making is valued. Train employees to work with data and understand the insights generated from machine learning models.
- Continuously Monitor and Optimize: Machine learning models are not static; they require ongoing monitoring and optimization to maintain their effectiveness. Regularly assess model performance and retrain with new data as necessary.
Case Studies: Success Stories in Business
Numerous companies have successfully integrated machine learning data models into their business operations, resulting in remarkable transformations. Here are a few examples:
1. Netflix
Netflix utilizes machine learning algorithms to analyze viewer patterns and preferences. By tailoring recommendations based on user behavior, Netflix has seen increased viewer engagement and subscription retention, proving the importance of personalized content delivery.
2. Amazon
Amazon employs machine learning across multiple functions, particularly in its recommendation engine. By analyzing customer purchase histories and browsing behaviors, Amazon can suggest products that customers are likely to buy, resulting in significant boosts in sales.
3. Uber
Uber uses machine learning models to optimize driver routing and pricing strategies. The algorithms analyze real-time data to determine demand patterns, ensuring passengers receive timely services while allowing drivers to maximize their earnings.
The Future of Machine Learning in Business
The future holds immense potential for businesses willing to embrace machine learning data models. As technology continues to evolve, we can anticipate advancements such as:
- Increased Automation: More processes will likely become automated, allowing businesses to operate with enhanced efficiency.
- Improved Machine Learning Models: As algorithms become more sophisticated, they will be able to provide more accurate and actionable insights.
- Greater Integration with IoT: The integration of machine learning with Internet of Things (IoT) devices can provide real-time data that significantly enhances decision-making processes.
Conclusion
As we have seen, the integration of machine learning data models into business processes is no longer optional; it is essential for achieving operational excellence and driving innovation. From enhancing content management services to streamlining business process automation services and reinforcing data governance systems, the applications of machine learning are vast and transformative. By leveraging these models effectively, businesses can not only optimize their current operations but also prepare for a prosperous future in an increasingly data-driven world.
Take Action Now
If your business hasn't yet adopted machine learning data models, consider exploring solutions that can enhance your operational processes. Engage with experts, invest in quality data, and foster a culture of innovation to see your business flourish.